« Previous 1 2
A new approach to more attractive histograms in Prometheus
Off the Chart
Prometheus histograms provide a method for displaying the distribution of continuous values. They provide information about the range and shape of the data and are often used to calculate percentiles. To do this, data is divided into 100 distribution areas. The x th percentile is then the value below which x percent of the observations fall.
The classical histogram metric divides a range of values into small sections ("buckets" in Prometheus) and counts the number of observations per area. In classical histograms, you first need to define these areas. Each range is represented by its upper limit. A range of 5s contains the number of all observations with a value less than or equal to five seconds. Besides the ranges, two other values are interesting: the sum total of all observations and the number of observations.
To illustrate, I'll look at a practical example wherein a histogram is defined with three buckets: 1s , 2.5s , and 5s . Two queries, one of which lasts two seconds and the other four seconds, are then observed. The first observation lies within the 2.5s , 5s , and +Inf ranges, and the second lies within the 5s and +Inf ranges (Figure 1). From this data, a histogram of HTTP request duration in seconds can be created in Prometheus (Listing 1).
Listing 1
HTTP Request Duration
# HELP http_request_duration_seconds Histogram of latencies for HTTP requests in seconds. # TYPE http_request_duration_seconds histogram http_request_duration_seconds_bucket{le="1"} 0 http_request_duration_seconds_bucket{le="2.5"} 1 http_request_duration_seconds_bucket{le="5"}
Buy this article as PDF
(incl. VAT)
Buy ADMIN Magazine
Subscribe to our ADMIN Newsletters
Subscribe to our Linux Newsletters
Find Linux and Open Source Jobs
Most Popular
Support Our Work
ADMIN content is made possible with support from readers like you. Please consider contributing when you've found an article to be beneficial.
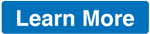