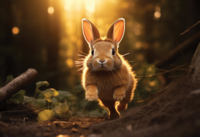
Lead Image © austler, 123RF.com
Kick-start your AI projects with Kubeflow
Quick Start
Artificial intelligence (AI) and machine learning have been on everyone's lips for some time now. The more accessible the technology becomes, the more developers will realize they need to develop algorithms that pervade the everyday lives of the masses and make life easier. However, interested developers initially face a challenge that has nothing to do with AI and language models: providing the technical infrastructure.
Kubeflow [1] is an open source machine learning platform that promises to offer all the tools you need for AI development, which means the components number well over 70, and they need to be rolled out and operated with the right configurations and in a correct and coordinated sequence. The enormity of this undertaking explains why more than a few developers feel they have been taken for a ride. Anyone who has never looked at Kubeflow will need more or less as much time to familiarize themselves with it as they would take to organize the required components themselves … and I've not even mentioned Kubernetes itself (see the "Taming the AI Infrastructure" box). Any administrator who has ever rolled out K8s knows that success is by no means guaranteed.
Taming the AI Infrastructure
Tools for building and training AI-based language models have been available for a long time. They often originate from the open source environment, which benefits from its proximity to academic circles and science when it comes to AI. A large number of the language models available today use open source scripting languages and libraries and are themselves published under free licenses.
In the context of machine-based learning, providing the technical infrastructure is no mean task. In fact, every AI model behaves like a complete program, to which components such as the material the
Buy this article as PDF
(incl. VAT)
Buy ADMIN Magazine
Subscribe to our ADMIN Newsletters
Subscribe to our Linux Newsletters
Find Linux and Open Source Jobs
Most Popular
Support Our Work
ADMIN content is made possible with support from readers like you. Please consider contributing when you've found an article to be beneficial.
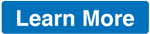