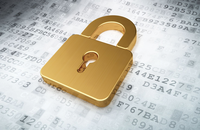
Lead Image © Maksim Kabakou, 123RF.com
Data security and data governance
New Gold
Data has a central function in the digital economy. It is collected in gigantic quantities so that automated or autonomous decisions can be made on the basis of data analysis. In this context, data security is achieved by technologies such as encryption, access protection, and tools that prevent attacks (e.g., SQL injection) on information. In contrast, data governance involves tools that encourage correct handling of data (e.g., managing personal and other sensitive information) and that implement the associated risk management with the use of, for example, metrics and control instruments.
The Basis: Data Overview
To implement data security, not just as a local solution for certain databases but to secure all or at least a large amount of relevant information, you first need to establish the basis. Data management informs you of the information you have, which is important because you can't use or protect what you don't know about.
And a positive side effect is that this knowledge is needed not only for data security, but also for the efficient use of data and for data governance. More specifically, it's about metadata management and data catalogs. Metadata management is the functional approach in data catalogs that acts as a repository of information across the various data storage systems. This helps you identify data worthy of protection, as well as the best data sources for the efficient and targeted use of information.
Data catalogs and metadata management have grown in importance, especially in the wake of the European Union General Data Protection Regulation (GDPR), with its stricter requirements for handling personal data. Some of the vendors in this market started with products for privacy management and then implemented data catalogs and metadata management as core components to serve requirements other than "simple" data protection.
The cost of data
...Buy this article as PDF
(incl. VAT)
Buy ADMIN Magazine
Subscribe to our ADMIN Newsletters
Subscribe to our Linux Newsletters
Find Linux and Open Source Jobs
Most Popular
Support Our Work
ADMIN content is made possible with support from readers like you. Please consider contributing when you've found an article to be beneficial.
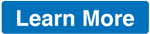