« Previous 1 2
Darshan I/O analysis for Deep Learning frameworks
Looking and Seeing
Summary
A small amount of work has taken place in the past characterizing or understanding the I/O patterns of DL frameworks. In this article, Darshan, a widely accepted I/O characterization tool rooted in the HPC and MPI world, was used to examine the I/O pattern of TensorFlow running a simple model on the CIFAR-10 dataset.
Deep Learning frameworks that use the Python language for training the model open a large number of files as part of the Python and TensorFlow startup. Currently, Darshan can only accommodate 1,024 files. As a result, the Python directory had to be excluded from the analysis, which could be a good thing, allowing Darshan to focus more on the training. However, it also means that Darshan can't capture all of the I/O used in running the training script.
With the simple CIFAR-10 training script, not much I/O took place overall. The dataset isn't large, so it can fit in GPU memory. The overall runtime was dominated by compute time. The small amount of I/O that was performed was almost all write operations, probably writing the checkpoints after every epoch.
I tried larger problems, but reading the data, even if it fit into GPU memory, led to exceeding the current 1,024-file limit. However, the current version of Darshan has shown that it can be used for I/O characterization of DL frameworks, albeit for small problems.
The developers of Darshan are working on updates to break the 1,024-file limit. Although Python postprocessing exists, the developers are rapidly updating that capability. Both developments will greatly help the DL community in using Darshan.
Infos
- Darshan: https://www.mcs.anl.gov/research/projects/darshan/
- Documentation for multiuser systems: https://www.mcs.anl.gov/research/projects/darshan/docs/darshan-runtime.html#_environment_preparation
- Darshan mailing list: https://lists.mcs.anl.gov/mailman/listinfo/darshan-users
- "Understanding I/O Patterns with strace, Part II" by Jeff Layton, https://www.admin-magazine.com/HPC/Articles/Tuning-I-O-Patterns-in-Fortran-90
- POSIX I/O functions: https://www.mkompf.com/cplus/posixlist.html
- Keras: https://keras.io/
- CIFAR-10 data: https://www.cs.toronto.edu/~kriz/cifar.html
- "How to Develop a CNN From Scratch for CIFAR-10 Photo Classification" by Jason Brownlee, accessed July 15, 2021: https://machinelearningmastery.com/how-to-develop-a-cnn-from-scratch-for-cifar-10-photo-classification/
- Anaconda Python: https://www.anaconda.com/products/individual
« Previous 1 2
Buy this article as PDF
(incl. VAT)
Buy ADMIN Magazine
Subscribe to our ADMIN Newsletters
Subscribe to our Linux Newsletters
Find Linux and Open Source Jobs
Most Popular
Support Our Work
ADMIN content is made possible with support from readers like you. Please consider contributing when you've found an article to be beneficial.
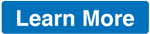