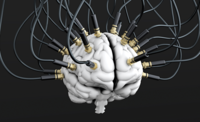
Lead Image © Maxim Basinski, 123RF.com
Artificial admin
Machine Brain
The innovation currently sweeping across the IT industry is artificial intelligence (AI). Language models such as ChatGPT, which can be trained with existing materials, have established themselves as a factor in our daily lives. However, language models are only a small part of AI and not exactly the most obvious candidates when it comes to integrating artificial intelligence into everyday IT processes. Instead, industry service Gartner listed better suited candidates a while back, while coining the term "AIOps" (artificial intelligence for IT).
After experiencing the pronouncement of DevOps, ChatOps, SecOps – and now AIOps – you might be frustrated by yet more stuff that mainly comes from the vendors' marketing departments and doesn't really address actual needs in the daily IT grind. What's more, when it comes to artificial intelligence, people are always worried about whether their jobs are at risk.
How real is this danger? Will computers soon take over the data center and completely replace admins, or has Gartner once again focused on a niche topic to grab attention? "No" to both questions; read on to find out why.
What's All the Fuss?
The one genuine problem in the context of discussions about AIOps is the vagueness of the term "AI." Like the cloud, AI has become part of the everyday lexicon, but it is used in a fuzzy way. Any process in which a computer is involved and that is not based on direct human input is already considered AI.
Gartner's understanding of AIOps, if you browse through the industry analyst's texts and flyers, is integrating artificial intelligence tools into everyday administrative work, and even partially replacing human skills in operations with AI-supported automation [1].
Some administrators might be surprised. For many years, monitoring systems such as Nagios or Icinga have offered options for providing automated responses to specific events, but this automation needed to be configured manually, and the results were often more damaging than the outcome they intended to prevent. Accordingly, this kind of functionality lies dormant in most setups today, and admins attach great importance to keeping it that way.
However, it is well worth taking a closer look at the theory behind Gartner's brand of AIOps and its implementation. Gartner quite rightly notes that IT has already changed massively in recent years and that artificial intelligence will sooner or later become a necessity, primarily because of an old acquaintance: the cloud.
Massive Setups
One forecast for the IT of the future seems certain: Small providers with rental space in any data centers will become increasingly rare. Instead, IT service providers will either specialize in individual services or mutate into platform providers themselves. Everything in between will disappear, leading to decreasing numbers of IT corporations grabbing a constantly increasing share of the overall IT market.
The focus on density and automation implicitly means fewer people in the control room responsible for increasing numbers of individual servers, which is unlikely to make their work easier; the larger a setup, all told, the larger its attack surface. In a small setup with low traffic levels, an admin might be able to tell whether a distributed denial of service (DDoS) attack is imminent by looking at the monitor with the RRD data of the installation in question. If they have to deal with thousands of servers and countless network devices, though, this strategy will no longer work.
Corporations that have already established themselves as platform providers have reacted to this scenario. Monitoring, alerting, and trending (MAT) are integral parts of large scalable platforms. Prometheus and VictoriaMetrics collect and consolidate metrics data, Grafana prepares and displays it graphically, and Loki takes care of the central collection of logfiles.
However, MAT is just a reaction to the ever larger and more complex setups that admins working for platform providers face. These tools theoretically enable an admin to identify attacks from metrics data, but, in practice, they would need to keep a constant eye on all of the platform's graphs and reliably identify even the smallest of changes. Neither the human eye nor the human brain can do that. This is exactly where the proponents of AIOps come into play.
The logic is simple: Where the human eye and brain fail, AI can easily do the job. The primary focus is not just on models for machine learning-based language skills, but on machine learning in general. Just as you can prepare an algorithm for the correct use of the language by feeding it language samples, you can use examples of real attack scenarios to teach a different kind of algorithm to identify the attacks at an early stage – and often in good time.
Almost every spam filter uses a very simple form of machine learning. However, the possibilities of artificial intelligence are far more comprehensive and already offer many more opportunities, which lead to even longer wishlists for the admins themselves – far more so for providers who look to keep admins happy with appropriately designed products while significantly boosting their revenues.
AIOps therefore needs to give companies a better response to attacks and administrative challenges in everyday life so that, ideally, dangerous situations cannot arise in the first place. As a result, the products used for this purpose will become cash cows for corporations such as IBM, Dynatrace, and others.
AIOps in Concrete Terms
Gartner might overuse the term AIOps without breathing life into it, but Red Hat and, in turn, IBM already have far more concrete ideas of how the practical benefits of AIOps could look.
In 2019, Red Hat presented an example that was based on the Prometheus time series database for predicting the probability of DDoS attacks from anomalies in traffic data (Figure 1). The design was relatively simple at the time: Prometheus, or more specifically its clustered form, Thanos, was used as the central element. Because Prometheus is really bad at storing long-term data, the setup was expanded to include Ceph, where the long-term data from Prometheus was moved. A setup comprising the Prophet and Fourier machine learning models then analyzed the long-term data and connected with Prometheus.
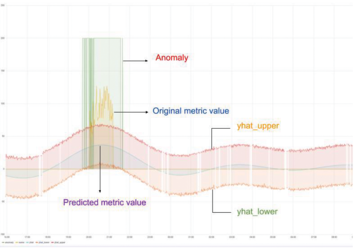
Prophet is a prediction environment developed by Facebook, whereas Fourier analyzes frequency information from traffic data streams and correlates the data with various additional environmental variables. The combination of Fourier and Prophet proved its value in this proof of concept. Feeding Prophet patterns of attacks that had taken place in the past meant that it gradually gained the ability to detect suspicious developments in the current data stream in just seconds.
Field tests show that a few seconds of unusual traffic are enough to predict an imminent attack with a high degree of reliability. Anyone who still claims that an attack can only be "predicted" once it is underway is wrong. The extremely practical combination of Fourier and Prophet not only identified the clear data patterns of attacks that had already begun but were also able to identify preparatory work (e.g., connections opened for reconnaissance of the environment) on the basis of various details of the individual connections.
Don't forget that Prometheus itself has a complete and well-maintained alerting engine. By notifying admins of impending attacks, the sample setup makes it possible to prevent the the attacks by reconfiguring the network and firewall (Figure 2).
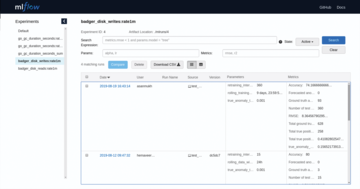
Corporate-speak around AIOps almost invariably means this specific form of machine learning from connection metadata. As IBM puts it in marketing jargon for Red Hat: AIOps is DevOps with big data.
Buy this article as PDF
(incl. VAT)