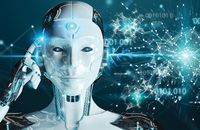
Lead Image © sdecoret, 123RF.com
Vector databases for data-driven artificial intelligence
New Dimensions
To remain competitive, companies in all sectors need to incorporate the principle of data-driven decisions into their business logic, bearing in mind that data growth, especially the growth of unstructured data, is omnipresent. Market research institutes assume significant growth rates in the global market for data-driven artificial intelligence (AI).
AI helps structure and manage the flood of data in a meaningful way – not just in large corporations, but also in small and medium-sized enterprises (SMEs). Well-designed AI-based applications rapidly search through even the largest of datasets to gain new insights and ultimately open new business models capable of generating real added value for companies.
The World of Complex Data
Against the background of these challenges, vector databases open a new category of database management and a paradigm shift in the utilization of the exponentially growing volumes of unstructured data that remain unused in object stores. Vector databases offer an amazing new range of opportunities, especially in searches for unstructured data; however, they can also process semi-structured and even structured data.
Unstructured data (e.g., images, videos, audio, or user behavior) generally does not lend itself to approaches that use legacy database models, where "legacy" means databases that use B-tree and hash indexes, which are not ideal for storing and retrieving high-dimensional and complex data. These databases seemingly suffer from the "curse of dimensionality," which makes them inefficient as the number of data dimensions increases.
Embeddings
Embeddings, generated by machine learning (ML) models to describe the essential features of data, overcome this curse of dimensionality, making it possible to represent complex data in a low-dimensional space and
...Buy this article as PDF
(incl. VAT)