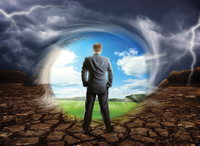
Lead Image © Sergei Tryapitsyn, 123RF.com
Apache Storm
Analyzing large volumes of data with Apache Storm
Huge amounts of data that are barely manageable are created in corporate environments every day. This data includes information from a variety of sources such as business metrics, network nodes, or social networking. Comprehensive real-time analysis and evaluation are required to ensure smooth operation and as a basis for business-critical decisions. A big data specialist such as Apache Storm is necessary to organize such amounts of data. In this article, I will walk you through the installation of a Storm cluster and touch on the subject of creating your own topologies.
Whether your company is in production or the service industry, the volumes of data that need to be processed keep growing from year to year. Today, many different sources deliver huge volumes of information to data centers and staff computers. Thus, the focus is on big data – a buzzword that seems to electrify the IT industry.
Big data concerns the economically meaningful production and use of relevant findings from qualitatively different and structurally highly diverse information. To make matters worse, this raw data is often subject to rapid change. Big data requires concepts, methods, technologies, IT architectures, and tools that companies can use to control this flood of information in a meaningful way.
Storm at a Glance
Storm was originally developed by Twitter and has been maintained under the aegis of the Apache Software Foundation since 2013. It is a scalable open source tool that focuses on real-time analysis of large amounts of data. Whereas Hadoop primarily relies on batch processing, Storm is a distributed, fault-tolerant system which – like Hadoop – specializes in processing very large amounts of data. However, the crucial difference lies in real-time processing.
Another feature is its high scalability: Storm uses Hadoop ZooKeeper for cluster coordination and is therefore highly scalable. Storm clusters are also easier to manage. Storm is designed so that all incoming information is processed. Topologies can, in principle, be defined in any programming language, although Storm typically uses Java.
A Storm environment usually consists of several components. A Storm cluster resembles a Hadoop cluster in many ways. Whereas MapReduce jobs are run in Hadoop, Storm uses the aforementioned topologies. Additionally, MapReduce operations are used by Hadoop for data consolidation; Storm again uses topologies. They are both very similar, except that MapReduce operations terminate on completion, whereas Storm constantly runs its topologies.
You will encounter two types of nodes in a Storm cluster: master and worker nodes (Figure 1). The Nimbus daemon, whose functionality is comparable to that of the JobTracker in the Hadoop environment, runs on the master node. Nimbus is responsible for distributing the code among the cluster nodes. It assigns tasks to the available nodes and monitors their accessibility and availability.
The supervisor daemon, which waits for work from Nimbus, runs on each worker node. Each worker process executes a topology subset. Conversely, this means that multiple worker processes (which are usually distributed over different computers) are responsible for executing a topology.
ZooKeeper is responsible for the liaison and coordination between Nimbus and the supervisor processes (Figure 2). The daemons are fail-fast systems and are therefore designed so that you can detect errors at an early stage and counter them. Because the daemon status information is managed in ZooKeeper, or alternatively on a local drive, they are extremely fail-safe. If the Nimbus or several supervisors fail, they are restarted automatically, as if nothing had happened.
Topologies and Streams
Distributed data processing is referred to as a topology in the Storm big data environment; it consists of streams, spouts, and bolts. Storm topologies are very similar to legacy batch mechanisms; however, unlike batch processing, they do not have a beginning and endpoint but instead keep running until they are finished.
The central data structure in Storm is the tuple, which – in simplified terms – consists of a list of key-value pairs. A stream consists of an unlimited number of tuples. The tuples are comparable to the events from the field of Complex Event Processing (CEP).
Spouts are the steam sources. You can understand them as adapters to the output sources, which convert the source data into tuples and then output these tuples as streams. Storm provides a simple API for implementing the spouts. Possible sources of data can include:
- Output of (network) sensors
- Social media feeds
- Click streams from web-based and mobile applications
- Application event logs
Because spouts do not typically use specific business logic, they can be used as often as you like in different topologies.
You can imagine topologies as a network of spouts and bolts. The bolts comprise the processing mechanism that receives the incoming streams, processes them, and then generates one or more output streams from them. Bolts can apply various actions to the incoming information. Typical functions can include:
- Filtering tuples
- Carrying out joins and aggregations
- Simple and complex calculations
- Reading and writing from/to databases
In principle, all processing steps are possible. Predefined topologies exist for typical processing steps; alternatively, adapting sample topologies is quite simple.
Setting Up Your Own Storm Cluster
Like Hadoop, Storm uses a typical master-slave environment but with slightly different semantics. In a classic master-slave system, the central server is usually fixed or set dynamically in the configuration. Storm uses a slightly different approach and is regarded as extremely fail-safe, thanks to the use of Apache ZooKeeper.
Storm is a Java-based environment, and all Storm demons are controlled by a Python file. Before actually installing Storm, you must first ensure that the necessary interpreters are correctly installed on the system concerned. You can also run the various Storm components on one system to familiarize yourself with them.
Storm was originally designed to run on Linux, but there are now also packages for Windows. Using an Ubuntu server is advisable if you want to evaluate Storm, because both Storm and the required components can be installed easily this way. If you are setting up a new Linux system, you should also select the OpenSSH server in the package selection.
The first step is the installation of Java components. The easiest way to obtain them is via the Apt package management system:
sudo apt-get update sudo apt-get --yes install openjdk-6-jdk
To start, the simplest way is set up a single-node pseudo-cluster on which ZooKeeper and the different Storm components can be run side by side. Storm requires the use of ZooKeeper from version 3.3.x onward. You can install the latest version 3.4.6 using the following command:
sudo apt-get --yes install zookeeper=3.4.6 zookeeperd=3.4.6
This command sets up the ZooKeeper binaries and the service scripts for starting and stopping ZooKeeper.
Next, you can turn to the actual installation of Storm. You will find the current archive on the Internet [1]. Start with the Storm users and the associated group. You can create this as follows:
sudo groupadd storm sudo useradd --gid storm --home /home/storm --create-home --shell /bin/bash storm
After downloading Storm, install the big data environment in the /usr/share
directory and create a symlink to the /usr/share/storm
directory. The advantage of this approach is that you can easily set up newer versions and only need to change a single symbolic link. Additionally, you can link the storm executables to /usr/bin/storm
:
sudo wget <Download-URL> sudo unzip -o apache-storm-0.9.2-incubating.zip -d /usr/share/ sudo ln -s /usr/share/apache-storm-0.9.2-incubating /usr/share/storm sudo ln -s /usr/share/storm/bin/storm /usr/bin/storm
Storm writes its logfiles in the /usr/share/storm/logs
directory by default instead of /var/log
, the default log directory for most Unix variants. To change this, create a subdirectory for Storm in which the log data can be written. To do so, enter the following commands:
sudo mkdir /var/log/storm sudo chown storm:storm /var/log/storm sudo sed -i 's/${storm.home}\/logs/\/var\/log\/storm/g' /usr/share/storm/log4j/storm.log.properties
Finally, move the Storm configuration file to /etc/storm
and create a symbolic link:
sudo mkdir /etc/storm sudo chown storm:storm /etc/storm sudo mv /usr/share/storm/conf/storm.yaml /etc/storm/ sudo ln -s /etc/storm/storm.yaml /usr/share/storm/conf/storm.yaml
This completes the installation of Storm, and you can now configure it and ensure the Storm daemon runs automatically.
Buy this article as PDF
(incl. VAT)
Buy ADMIN Magazine
Subscribe to our ADMIN Newsletters
Subscribe to our Linux Newsletters
Find Linux and Open Source Jobs
Most Popular
Support Our Work
ADMIN content is made possible with support from readers like you. Please consider contributing when you've found an article to be beneficial.
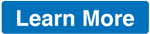